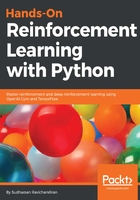
上QQ阅读APP看书,第一时间看更新
Agent environment interface
Agents are the software agents that perform actions, At, at a time, t, to move from one state, St, to another state St+1. Based on actions, agents receive a numerical reward, R, from the environment. Ultimately, RL is all about finding the optimal actions that will increase the numerical reward:

Let us understand the concept of RL with a maze game:

The objective of a maze is to reach the destination without getting stuck on the obstacles. Here's the workflow:
- The agent is the one who travels through the maze, which is our software program/ RL algorithm
- The environment is the maze
- The state is the position in a maze that the agent currently resides in
- An agent performs an action by moving from one state to another
- An agent receives a positive reward when its action doesn't get stuck on any obstacle and receives a negative reward when its action gets stuck on obstacles so it cannot reach the destination
- The goal is to clear the maze and reach the destination